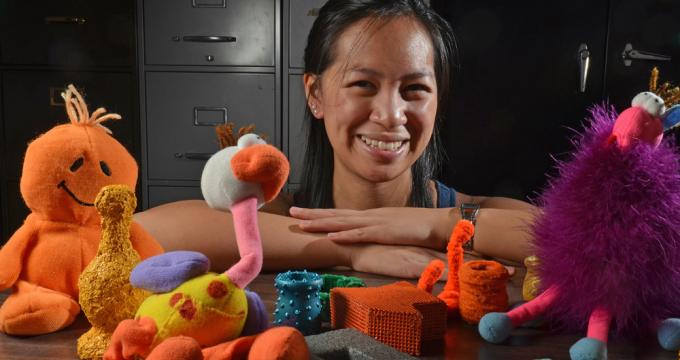
Lead by Professor Eliana Colunga, the CU Language Project aims to understand “how young children learn language” and, currently, the lab's main line of research looks at the relationship between a child's existing vocabulary and the way he or she acquires new words (CU Language Project). As part of one such inquiry, Professor Colunga's lab is collecting snapshots of children's vocabularies at different ages and, drawing from participants that acquire language at different rates, the team wants to discover what allows some children to learn language children show when learning a novel word in the lab (i.e., shape and material biases) in addition to a word's shared features including semantic features (meaning), phonological features (sound properties), logical associations, co-ocurrences (words said together), etc. Together, these different types of connections between words will help researchers understand how children learn language and, ultimately, how to improve modeling of language acquisition (Radford).
While the team has attempted to visualize their data to gain the high level intuition necessary for identifying promising trends and possible models, Professor Colunga and her researchers have expressed frustration with standard scientific visualization packages like JUNG. Specifically, drawing upon preliminary semi-structured interviews, the lab has failed to produce graphics that simultaneously convey the data's longitudinal, multivariate, and layered nature, leaving them to explore limited subsets at a time. On the other hand, recent strides in the data visualization community have expanded upon methods for exploring highly dimensional and changing data sets like those from the CU Language Project. Leveraging layered informationPottinger 2 displays, carefully constructed "small multiples” for comparisons, and novel representations of networks, modern data visualization techniques could likely reveal new insights in the lab's data (Tufte, Envisioning Information; McLachlan, Munzner, Koutsofios, and North, "LiveRAC"; Munzner, "Portfolio"). Still, as prolific visualization researcher Tamara Munzner observes, many disciplines like Professor Colunga's still require "problem characterization and abstraction" before appropriate visualizations can be generated (Michael Sedlmair, Miriah Meyer, and Tamara Munzner, "Methodology" 2432). Thus, given the team's requirements for exploring a multivariate and dynamic problem domain, the slim visualization literature characterizing longitudinal vocabulary data, and recent relevant strides in the visualization field, this thesis contributes an abstraction of these domain-specific problems, provide an initial exploration of a design solution, and evaluate that solution using methods standard to data visualization research.